Rooting Out Sepsis Using Artificial Intelligence in the Emergency Department
Sponsored by Sepsis Alliance
Date: March 23, 2023
Speaker:
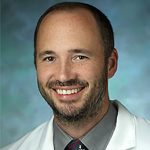
Jeremiah Hinson, MD, PhD
Associate Professor of Emergency Medicine, Associate Director of
Research for the Department of Emergency Medicine
Johns Hopkins School of Medicine
Rapid advancements in artificial intelligence (AI) in healthcare are significantly transforming the practice of medicine by enabling higher levels of efficiency and speed, care delivery, and confidence in decision making. During this presentation, participants will learn about emerging AI technology and its potential to revolutionize our approach to sepsis care by enabling rapid triaging and disposition of patients at risk for or suspected of having sepsis. AI-driven tools that support improved sepsis detection, diagnosis, and treatment will also be discussed. The presenter will review recently published scientific literature on this topic and provide a framework for understanding these tools and integrating them into clinical practice to achieve widespread adoption and deliver maximum impact.
Speaker | Time Stamp | Speech |
Announcer – Hannah Eichelberger, Sepsis Alliance | (00:00) | Hello everyone. Welcome to our webinar, Rooting Out Sepsis Using Artificial Intelligence in the Emergency Department. Sepsis Alliance would like to gratefully acknowledge the support provided for this webinar by Beckman Coulter. The content in this presentation is determined by the sponsor. And just as a reminder, Sepsis Alliance's mission is to save lives and reduce suffering by improving sepsis awareness and care, both for the public and for healthcare professionals. And the information presented in today's webinar is intended for educational purposes only. |
Announcer – Hannah Echelberger | (00:34) | So with that, I'm so excited to introduce our presenter, Dr. Jeremiah Hinson. Dr. Hinson is an Associate Professor of Emergency Medicine as well as the Associate Director of Research for the Department of Emergency Medicine at the Johns Hopkins School of Medicine. Dr. Hinson, thanks so much for being here and I'll now turn it over to you. |
Dr. Jeremiah Hinson, Johns Hopkins School of Medicine | (00:54) | Awesome, thank you Hannah. I'm going to share slides for my presentation. Hopefully everyone is seeing it now. |
Hannah | (01:09) | Yep, looks good. Thanks. |
Dr. Jeremiah Hinson | (01:10) | Perfect. All right, thank you. So like Hannah said, I'm from the Department of Emergency Medicine at Johns Hopkins. We're going to put up a couple more polls if folks could answer those will be helpful in terms of thinking about artificial intelligence in general and how particular tools may apply to the folks in the group here. We'll kind of cycle through these polls as we're doing the introduction. I think Hannah will take care of that. |
Dr. Jeremiah Hinson | (01:40) | Before we start, let me see if I can advance. Yeah, so just want to put a disclosure up here. So at Johns Hopkins, almost a decade ago, we founded a small startup company with the support of the National Science Foundation called Stocastic Johns Hopkins Startup Company. I worked as the Chief Medical Officer for this company and our goal was to take products that we developed through research using usually federal funding and disseminate them nationally so that more people can use them through commercial pathways. Within the past year, that company was purchased by Beckman Coulter and I am continuing to work on some of those products with Beckman Coulter as a consultant. And so we'll talk about some of them during this presentation. |
Dr. Jeremiah Hinson | (02:30) | So we're here to talk about sepsis and I think the fact that you're here listening to the presentation means that we agree sepsis is a major problem. Every time I talk about sepsis, I kind of like to talk about these statistics because they are so shocking. So a quarter million Americans die from sepsis each year. We've put a ton of time money into studying it, and this is still where we are. Across the globe, there are up to 3 million annual cases of sepsis. The mortality rate has stayed pretty steady at 15% to 30% for folks who get sepsis. And then of all the patients who die in the hospital, up to half of them are dying from sepsis. More than 10% of all of our admissions to ICUs are for sepsis. And we know that early diagnosis and treatment is really critical to survival. |
Dr. Jeremiah Hinson | (03:19) | So we've done a lot of work including clinical trials of which I've been part of looking for new therapies for sepsis. But the mainstay is still really antibiotics and hemodynamic stabilization using fluids and vasopressors. But we know that implementation of these very simple therapies has to happen early for the patients to have a good outcome. |
Dr. Jeremiah Hinson | (03:39) | This is where I work, this is Johns Hopkins Hospital. On the upper left hand corner is our old historic building. Our emergency department is actually in the new wing of the hospital, which is pictured kind of in the back there on that picture, but also in the picture on the right. We see a ton of patients, we sit inside the city of Baltimore, so we take care of a wide array of conditions and this is picture of our actual emergency department. This is before we had patients in it when it was being built. |
Dr. Jeremiah Hinson | (04:06) | If you went in today, it's much more chaotic, people everywhere, people scurrying around and trying to deliver care. And the reason I'm opening with this is just to point out that in emergency departments like this across the country, we really are the frontline for all types of care and sepsis care in particular. So two thirds to three quarters of all patients who come into the hospital are first seen here. |
Dr. Jeremiah Hinson | (04:28) | So when we start thinking about how we can intervene early, this is one of the most important places to work, inside the emergency department. I'm fortunate that at Johns Hopkins I get the privilege to work with a bunch of really talented and intelligent people in this Center for Data Science and Emergency Medicine. So we have colleagues from the Schools of Medicine, School of Engineering, School of Public Health, and then even from the Maryland Institute College of Art, who have collaborated on a lot of the work that I'll show you today. |
Dr. Jeremiah Hinson | (05:01) | And so it's worked by a big group of people - engineers, physicians, designers - that get us to the end products that we're talking about. And we've been funded by a lot of organizations, many of which are pictured here. So just a word of thanks to all the people that contributed to this. |
Dr. Jeremiah Hinson | (05:17) | When we talk about trying to support decisions and improve outcomes for patients, I think it's useful to kind of break down the decisions that are made in an environment like the emergency department into buckets. And these four buckets make good sense. So the first set of decisions we make, is actually a decision made mostly by nurses. And I see we have a lot of nurses on today, which is great to have you here. It’s the decision of triage. So there's a patient who just arrived at the hospital - how sick are they and how quickly do we need to see them? |
Dr. Jeremiah Hinson | (05:48) | The second set of decisions we start making is about diagnosis. Not like what does the patient have? That is one of those decisions, but also which diagnostic tests should I order? Once the results start coming back, how do I interpret them and how do I apply them to this specific patient? |
Dr. Jeremiah Hinson | (06:04) | Our next set of decisions is about treatments and sometimes those decisions have to be made before we even have the diagnosis. So there are therapies that we give that are supportive. So for a patient who has low blood pressure, we're giving fluids, maybe even vasopressors before we know exactly what is driving that low blood pressure. |
Dr. Jeremiah Hinson | (06:21) | And then there are treatment decisions we make about specific disease states. So for a patient with infection, we may give antibiotics and they can be broad spectrum or narrow depending on what we think that patient has. |
Dr. Jeremiah Hinson | (06:33) | And then perhaps the most important decision that we make in emergency medicine is something called disposition. It's really just a fancy word for where should this patient go? Is this a patient that we have stabilized and they are safe to go home and follow up with a primary care doctor? Do they need to come into the hospital? If they do, are they going to be here for less than 24 hours and we should just observe them versus admit them? Do they need to go to ICU? Do they need to go to a Step Down Unit? And all of these decisions are being made within a span of about six hours, hopefully, for a single patient, but they're being made by one physician for multiple patients all at one time. |
Dr. Jeremiah Hinson | (07:08) | So the emergency department is actually probably the most hazardous decision making environment in all of emergency medicine. It's where the most decisions are made in the smallest amount of time. So I'll kind of go through these five problems that we're trying to address, both through decision support and other tools to make things safer in the emergency department. So the first is overcrowding. So this is a report from the Institute of Medicine in 2007 basically saying “Emergency departments are so crowded, if we don't address this, the entire health system's going to implode.” And since 2007, things have not improved. They've actually gotten worse in terms of hospital emergency system crowding. We're also very frequently interrupted in emergency medicine because we're caring for so many different patients. Some of them are critical, some of them are stable. And we're asked to task switch, like move from one thing to another every three to five minutes. And there are a lot of studies actually measuring this and showing that. |
Dr. Jeremiah Hinson | (08:06) | And then the EHR, as we'll talk about in a moment, the Electronic Health Record has been a huge advancement in medicine, there are really good things about it, but it also pulls the clinician away from direct interactions with other members of the healthcare team and direct interactions with the patient can make decisions more difficult. |
Dr. Jeremiah Hinson | (08:24) | Over the same decade, another change that has happened is the architecture and environment that we work in. If you went into an emergency department 15 years ago, you would stand in the middle, see all patients at one time. And now in newer emergency departments including those I work in, all those patients are behind doors. And that's done to optimize patient privacy, which is very good and important, but it also can be a challenge to patient safety and decision making because you can't see those patients all the time and relying on monitors and things like that. And then last, we practice in an environment that's unique in that we rarely get to learn from the decisions that we make and in real time. |
Dr. Jeremiah Hinson | (09:02) | So in an ideal system, you make a decision, there's an outcome from that decision and you learn, “Was that a good decision or a bad decision?,” and it informs your next (decision). In emergency medicine, we often see patients that we have no relationship with, we don't see them again, we don't know what happens to them, whether they're discharged or hospitalized. And so it can make it difficult to exercise practice-based learning. We call that an open loop learning system. |
Dr. Jeremiah Hinson | (09:25) | So more about the Electronic Health Record, because it's really where we're going to focus. So it has really transformed the way that information is gathered and patient care is delivered. In 2010, when I started emergency medicine, we were gathering all information on pieces of paper here that could be completed really quickly. And now one decade after that, everything is in an Electronic Health Record. We're capturing tons of data. We can look at a ton of data. Really changes the way we approach the patient encounter. |
Dr. Jeremiah Hinson | (09:53) | And it has totally revolutionized the way that we store health data and how usable those data are. So if you look back in New England Journal of Medicine a couple decades ago, you would see very small studies that were getting published in high tier journals. And that's because we didn't have access to enough data quickly to study very large populations. |
Dr. Jeremiah Hinson | (10:12) | Now we have whole warehouses full of data. Every event that occurs in the hospital is captured in some way and we have the potential to use that data to inform future decisions. So some questions that I'm hoping to address today by going through specific examples are these: So can we use Electronic Health Record data and artificial intelligence together and harness those for clinical purposes? Can those tools outperform current standards and can they be translated to clinical practice? And this last question is a challenging one, often the most challenging one. So we'll spend some time on that and then I'm going to do that by using one example from each of these buckets that we talked about earlier: Triage diagnosis, treatment and disposition. |
Dr. Jeremiah Hinson | (11:03) | So we'll start with triage. So again, triage is this first decision that's made, a patient arrives to the emergency department. They often visit a triage booth or desk where a nurse is and they talk about why they're there and we decide what to do. So why do we bother triaging? Because this is the common scenario, right? You have a 40 bed emergency department, you have three doctors available and you have 80 patients. So you can't see them all. Which patients should be seen next? Are there patients among those presenting who really cannot wait? If so, you have to prioritize them. And then you only have 80 beds. The likelihood of being able to see everybody in one of those beds is low. So are there patients who can be taken care of in more creative ways, like maybe in chairs or a hallway, folks who don't necessarily need a bed? |
Dr. Jeremiah Hinson | (11:52) | And so those are some of the questions that we're trying to address with triage in the emergency department. The gold standard tool is the Emergency Severity Index, and it's pictured over here on the left hand side of our screen. It is a pretty simple triage scale that was developed in 1999, came out from AHRQ and a group of investigators and it assigns patients to levels 1-5 using some very simple criteria, many of which are clinician intuition. |
Dr. Jeremiah Hinson | (12:25) | So is this patient who's dying? If so, they need to be seen right away. They're a level one. Is this a patient who shouldn't wait, they're not safe to wait? If so they're assigned to level two. And those patients in buckets one and two are seen right away. They have definitive care pathway. And then we move on to how many resources is the patient anticipated to use and we count those and they could be assigned to level four, five or to three. |
Dr. Jeremiah Hinson | (12:50) | So patients who go to level four or five, they often have a parallel pathway within emergency departments where they're seen in a different area like a urgent care or fast track. And they'll often be seen by even non-emergency medicine-board-certified positions: physician assistants, NPs, some of our allied health providers. And then the third bucket is this level three. So the trajectory is really uncertain. Some of these patients are sick, some of them are not sick, and they are competing for beds with these levels, level one and twos. |
Dr. Jeremiah Hinson | (13:24) | What you would want is to have very few people in an uncertain bucket after you perform triage. But what we see is actually at Johns Hopkins when we began this and then nationally the same thing is true, the majority of patients go to this middle tier bucket three level of uncertainty. And a lot of time is spent trying to re-triage those patients and determine which of those patients do we need to pull back next from the level threes in the waiting room. |
Dr. Jeremiah Hinson | (13:49) | We call that three-iage. The process of triaging the level threes. We've done a ton of work in this space and these are two articles that we've actually published about triage in the Annals of Emergency Medicine. We see that between two nurses using ESI, there's a 60% agreement and this was reproduced in multiple countries in different health systems in this study. So a little better than a flip of a coin between two different triage levels. |
Dr. Jeremiah Hinson | (14:21) | We also see, regardless of whether you're using ESI or some of the other tools like the Canadian tool or the Manchester tool, there's very low sensitivity for critical illness. So for example, one study using ESI found that in elderly patients who required an immediate lifesaving intervention in the ED, and this would be things like intubation, cardioversion, CPR, these patients, one in five of them was assigned to level three. |
Dr. Jeremiah Hinson | (14:50) | And remember that's that uncertain level that can be sitting in the waiting room for a prolonged period of time. So pretty low sensitivity for that. This is a great article, hot off the press. This was just published by a different group out of Kaiser Permanente. Dr. Sax led this investigation. It's a really good article. I encouraged people to read it. They looked at over 5 million patients throughout their health system nationally and they just looked at how comparing what actually happened to the patient and what their triage level was assigned, they found that 32% of patients were mistriaged. They found that over triages more common than under triaged, but under triage did occur, which can be dangerous. And then the sensitivity for patients requiring immediate lifesaving intervention was around 66% overall. So just adding more evidence to the fact that triage is a really challenging thing to do. |
Dr. Jeremiah Hinson | (15:44) | So it's challenging to predict what's going to happen to a patient when you're seeing them. And I should point out that triage occurs under a lot of time pressure. So there's often a line of patients arriving and these nurses are being asked to make these assignments very, very rapidly. So it's understandably challenging. |
Dr. Jeremiah Hinson | (16:04) | So what we did at Hopkins, and this is work that has occurred over the past eight or so years, we developed a tool that supports nurses in the triage decision, doesn't replace them, but uses predictive technology, artificial intelligence, and in this case machine learning models to support the decision. And I'll talk a bit about how this works and then how this plays into sepsis. |
Dr. Jeremiah Hinson | (16:28) | So we developed tools and this was led by Scott Levin, a colleague of mine, and he built machine learning models that can take the same information that is gathered already for ESI. And those are the chief complaint, the vital signs at arrival, demographics, how they came, whether in an ambulance or car. And then medical history from the Electronic Health Record. It's put into a machine learning model and that machine learning model is based on all of the encounters that exist in the Electronic Health Record at that local facility previously. So hundreds of thousands of encounters can predict the likelihood that the patient will go on to have one of three outcomes. |
Dr. Jeremiah Hinson | (17:07) | So a critical care outcome, needing to go to an ICU or dying an emergency procedure like a cardiac cath or an emergency endoscopy for bleeding, or needing hospital admission. Those probabilities are percent chance of something happening are then used to generate recommended triage acuity levels. And so you can see that in the middle figure here where we have a level 1, 2, 3, 4 or 5 being recommended based on risk threshold cutoffs that are established in collaboration with the local leaders of a hospital. |
Dr. Jeremiah Hinson | (17:43) | And what we see is that by moving these thresholds up or down, we can drive different distributions to different triage levels. And so this is an example from the Johns Hopkins Health System where the gray line in the middle here on the far right represents the time when we implemented this electronic triage, knock off TriageGO previously referred to as E-triage, but we're driving down the number of patients that were put into that middle tier uncertain level three bucket. And we do this by driving up the number of low acuity patients and we're able to care for these people in our fast track area. And I'll talk to you about this, kind of the safety profile of doing this, which is very good and how it impacts operations within the emergency department. |
Dr. Jeremiah Hinson | (18:30) | So how does it work in clinical practice? Well, in clinical practice it's very non-disruptive, we designed it this way. So a patient arrives here to the triage nurse, data are gathered, they're the exact same data that were gathered previously when the nurses were using ESI, they entered in the exact same place that they would when they're using ESI. |
Dr. Jeremiah Hinson | (18:52) | But in the two seconds that it takes to advance from one screen to the next, those data are fed to a cloud and then they are processed by the machine learning algorithms in the cloud and the tool puts decision support back into the Electronic Health Record. And it just appears when you advance the next screen. And you can see here that TriageGO makes a recommendation around the triage acuity. |
Dr. Jeremiah Hinson | (19:20) | It then includes some information about why a particular triage level has been recommended and then the nurse can choose to use that recommendation and follow it. Or sometimes the nurse may be seeing something that the machine is not able to see and it's not recorded in the Electronic Health Record and can override it and make a different decision. So the nurse who's making these decisions is not being replaced but rather being supported with objective data based on prior encounters at an individual facility. |
Dr. Jeremiah Hinson | (19:52) | So what happens when we put this in? Well, a lot of things. So at our own facility, we have begun to actually announce all level twos over a loudspeaker because we know that now the level two assignment is much more reliable. Those folks are likely to go on to have critical outcomes. And what we were able to do was drive changes in the way they flow so that we get them to the right place faster. So once we put in TriageGO, we saw a 52% improvement in capturing them as level one or two. |
Dr. Jeremiah Hinson | (20:30) | So patients who were going to critical care, we had 120% improvement in the capture rate as level one or two of patients who went on to have an emergency procedure. And then in the patients that we labeled low risk, so level four or five, we actually saw the rate of hospitalization in this group going down. |
Dr. Jeremiah Hinson | (20:50) | So not only are we changing those distributions like I showed you on the last slide, we're more accurate in our triage level assignment in terms of what's happening to the patients after. And this changes things. So if you have those patients who are going to the ICU no longer competing with people who are not as sick for beds, they get to the beds faster and decisions can be made faster. And we saw that we're able to get patients to the ICU or to an emergent procedure an hour faster in our system. And this has been true in other places where we have implemented it as well. |
Dr. Jeremiah Hinson | (21:23) | And then for the total population of ED patients, we decrease the door-to-disposition decision by almost a half hour. And this can really unlock a lot of capacity and cost savings. These are some estimates here. So up to 10,000 bed hours gained for an average emergency department and about a half a million dollars in cost savings in a year. And so the performance of the algorithm has been really consistent. Numerous hospitals across the country used it in more than a million and a half encounters now. |
Dr. Jeremiah Hinson | (21:55) | So I'm going to move on, we'll talk a little bit more about TriageGo in a second, but I'm going to move to the decision of diagnosis and we'll stick with the theme of sepsis, right? So we have a lot of patients in the emergency department, very few of those patients have sepsis, but we're very worried about it. So how do you identify which patients have sepsis and how do you get the intervention to the right people at the right time? Sepsis is particularly challenging. As you can see here there have been three sets of definitions for sepsis. |
Dr. Jeremiah Hinson | (22:25) | So even at the highest level, we're having a hard time agreeing about what sepsis actually is and how we define it. This is some screenshot of articles in the same issue of critical care where people are sort of having differing opinions about commonly used screening tools, qSOFA and SIRS, and how we should use them both in defining and screening for sepsis. So there's no argument about the fact that we all want to take better care of patients with sepsis. There's also no argument about the fact that it's hard to do it. It's hard to even define sepsis and identify the patient when they're right in front of us. |
Dr. Jeremiah Hinson | (23:06) | So in this slide, this is actually an article that's not from our group. I'm going to go through a few really good studies from other groups throughout this as well. This was done by a group out of Tenet Healthcare led by Spencer Jones. And they asked the question, "Okay, can we move beyond some of these commonly used screening tools? Can we develop a machine learning tool, a tool that uses artificial intelligence to identify patients with sepsis in the emergency department? And would it do better than the commonly used tools?" So before we get into the data in the table, I ask you look at the top right here. For folks not accustomed to looking at receiver or receiver operating characteristic curves in the area under them, it's just important to know that we grade these on a scale of 0.5 or zero actually, but all the way up to 100%. So 1.0. |
Dr. Jeremiah Hinson | (23:56) | And this is kind of how we rate them. When you look at the curve itself, the more area that is under the curve, the better it is. So obviously in this picture, the red curve is by far the best curve and that curve happens to correspond to the machine learning model that was developed by Dr. Jones and his team. And it's a lot better, it's a lot better than SIRS, it's a lot better than qSOFA, SOFA NEWS or MEWS, which are these three ladder scoring systems are more complicated illness severity scoring systems that are challenging for us to actually calculate during an emergency department stay. |
Dr. Jeremiah Hinson | (24:31) | But he compared against those and still outperformed them. And these are the actual sensitivity, specificity and area under the receiver operator curve, values that he and his group were able to achieve. So the take home here is that, yeah, machine learning can definitely beat all of the other sepsis screening tools and it can do it by a long shot. |
Dr. Jeremiah Hinson | (26:35) | So just a point to say that these tools have a lot of value, they have a lot of potential, but we have to be very careful in how we implement them. So this is a study published in JAMA looking at a model that was developed in one location and then implemented widely in other locations. The performance in the original derivation cohort where they developed it was very good. It looked very promising. And so the decision was made to roll this out widely and those of us who may have used this tool or similar tools have noticed that the performance was not what we would expect. |
Dr. Jeremiah Hinson | (27:15) | And so some folks at Michigan actually went and studied in their population how well it was performing and they found that the AUC, the area under the receiver operator curve was only 0.63, which is pretty poor. Alerts for this tool were firing on one in five patients. Out of every patient that got an alert, out of every eight patients only one of them had sepsis and the sensitivity was only 33%. So despite all of these alerts, two-thirds of the patients who had sepsis were not being recognized by the tool. |
Dr. Jeremiah Hinson | (27:47): | So the tool has been reworked and is doing a bit better now, I don't know what the new performance standards are, I don't think they have been reported, but it was a big kind of shock to the community that a tool like this would not work as well afterward as it had been reported initially. |
Dr. Jeremiah Hinson | (28:02) | Well, there's some key takeaways that we have here. So model transportability is not guaranteed. So when we talk about external validity and things like that, and when you're using simple clinical decision rules, it's straightforward that you need to externally validate. But it's even more important with machine learning tools because these tools use data that has been stored in the Electronic Health Record. And not only can the population be different, but you can also have big differences in the way data are stored or even gathered in the health system that can affect the model. |
Dr. Jeremiah Hinson | (28:33) | So you really need to know how well you're performing at your local site before you just go live with a tool. And so that kind of leads into the next. So we need to have transparency and oversight with these tools so that the people who are implementing a tool like this have to know how a tool was developed, how it was trained, and then know how it's performing at their particular site before it gets deployed. And then once something has been deployed, it's really important that we have very good systems in place for monitoring performance. And if the performance is not what we would expect, we need to figure out why that is and fix it or pull the tool. |
Dr. Jeremiah Hinson | (29:08) | And so I think because of this and many other things, regulation's really the future. So the FDA has already stepped into this space and is going to be regulating diagnostics that use artificial intelligence to make specific diagnoses. And that's going to be governed under software as a medical device. FDA's sort of new in this area, so a lot of things are under development and whether we'll have only locked models or models that can be optimized for specific locations is, I think, yet to be determined and a lot will develop here in the next decade. |
Dr. Jeremiah Hinson | (29:42) | So we'll move to treatment. And for treatment, I'm going to talk about some next generation things that are really exciting but not necessarily in standard practice yet. So the first of these is heterogeneity, right? So if you just walk into an emergency department or hospital in general, you walk from room to room, the patients don't look like each other, they're not the same age, they're not from the same place, they don't have the same types of past medical history and beyond that, genetically may just be very different. |
Dr. Jeremiah Hinson | (30:11) | So for a disease like sepsis, we have traditionally, and all clinical trials that have been performed to date, have treated everyone the same. So everybody gets the same therapy regardless of who you are. But we're starting to learn more and more that gene expression patterns can be different in response to different diseases and may change the way we choose to treat someone. This is true for sepsis, it's also true for different types of cancers. In cancer we've been a little more successful in identifying some of these. But in the future I think we're going to do a lot more of this where we try to understand a genetic profile prior to initiating therapies. And I'll show you some examples of early stage things that are moving toward practice at this point. |
Dr. Jeremiah Hinson | (30:52) | So these are two articles with some complex looking figures, and I don't think we need to go into too much detail about these except to say if you're interested in this area, these are two great teams who have studied this and it's worth reading them. |
Dr. Jeremiah Hinson | (31:07) | But basically what these folks did is they took big populations of patients with sepsis that are manifesting things in different ways. So they all have sepsis, but they all look very different. And they perform genetic analysis and applied types of machine learning to these, unsupervised cluster analysis to identify patients who looked similarly. And there've been at least three groups who have done this. And all three of these groups have come up with similar clusters. So some patients who are adaptive and tend to have a good outcome no matter what, patients who are inflammopathic don't have as good of an outcome. And patients who are coagulopathic tend to have very poor outcomes. In those sorts of manifestations of disease, they go back to their genetics and the genes that are being expressed and they can actually identify specific profiles that can be known upfront using these advanced molecular testing. |
Dr. Jeremiah Hinson | (32:08) | This next article is similar except they took a different approach, rather than using molecular techniques and sequencing transcriptomes, they just looked at data. 29 variables that are routinely available in the Electronic Health Record. The fanciest most exotic piece of data that they used here was c-reactive protein and ESR, things that are very routinely available. And they found very similar clusters. And what this showed was that yes, this is a super important concept, we're showing that it has validity at the genetic level, but you can just look at the phenotype of the patient in front of you in the emergency department. And by applying artificial intelligence to those EHR data, you can find similar trends. |
Dr. Jeremiah Hinson | (32:53) | And then the second take home from this article, which is maybe the coolest one, is that they went back and looked at data from sepsis trials. There have been over a hundred trials on sepsis looking for a new therapy, and none of the trials that have been done rigorously have found anything that worked. But maybe that's because we're putting the wrong people into the studies. |
Dr. Jeremiah Hinson | (33:14) | So if they changed the composure or the composition of the trial participants based on their type here, either alpha, gamma, beta and delta, they could move a trial from being a negative trial to a positive trial or to a trial that showed actual harm. And so maybe the reason we're not seeing any change in our treatments with sepsis is because we're treating everybody with sepsis the same and they're really not the same. So I think this will be the kind of way of the future is when we really extend personalized medicine to all diseases including sepsis. |
Dr. Jeremiah Hinson | (33:52) | So I'm going to end with one example here of how we can use artificial intelligence and clinical decision support to help us with that final decision, where should the patient go? All right, so I'm going to move to a different type of sepsis for this one. So this will be viral sepsis, and I take you back a couple years to the beginning of the Covid-19 pandemic. For those who are here and who were working in healthcare at this time, it was a pretty scary time. |
Dr. Jeremiah Hinson | (34:18) | There was this new condition that was very contagious. And more importantly, when you're trying to care for a patient, we didn't know which of these patients are going to get sicker, which are going to be stable and which are going to be just totally fine and get better quickly. And then in addition to the uncertainty at the individual patient level, we did not know what was going to happen at the population level. These are real predictions that were being made at the time. We either thought there was going to be an ebb and flow of patients, there was going to be a huge massive peak of patients, or we'd have the initial peak that we'd have and then not really see any more patients. |
Dr. Jeremiah Hinson | (34:55) | And so in this setting of uncertainty, leaders of the Johns Hopkins Health System reached out to our team based on work we'd done with the triage tool and asked us if we could develop something to put in place to help clinicians actually make better decisions about the disposition for patients right away. And this has been published as well. This is actually in Nature Digital Medicine Journal, so if you want to see more details about that or any of the work that we're talking about, almost all of this work is published and can be seen. |
Dr. Jeremiah Hinson | (35:26) | So because we were already working on some other things, we were able to rapidly develop an end-to-end artificial intelligence driven decision support system in around three months that was specifically tailored for this problem. So we curated a huge amount of data, all the data we had available for Covid-19 and across five hospitals in the Baltimore Washington region. We developed and tested these machine learning models and then we were able to hook them into our cloud-based technology and get clinical decision support into the Electronic Health Record and co-design that with physicians that were working at the time. |
Dr. Jeremiah Hinson | (36:08) | So this is how it worked. This is kind of a complex illustration, I walk you through it, we don't need to spend too much time. But one thing I want to say here is that all of this level of explanation was actually available to clinicians in real time and they could dig into the tool as much as they wanted to understand exactly how it was working. |
Dr. Jeremiah Hinson | (36:27) | Essentially, we used similar predictors to what the triage tool uses, but we added other things to it. So what are the vital signs in the emergency department for a particular patient? How have they been trending, are they getting better or worse? How much oxygen and what type are they using and what have their lab results been? And we looked for specific labs that were important for this prediction rather than just using all. |
Dr. Jeremiah Hinson | (36:51) | We then developed two different machine learning algorithms. So one of those algorithms predicted likelihood of a critical outcome within 24 hours, and we defined it as here, death, respiratory failure, cardiovascular failure or admission to a Step-Down or ICU. And then we also wanted to see which patients would develop a 72 hour inpatient care needs. And so early on in the pandemic, a lot of patients were being admitted. So we had good data on them and knew what would happen to them for the next 72 hours, but we had too many patients being admitted and it was kind of overwhelming our health system. So we looked for very specific markers of cardiovascular and respiratory dysfunction that indicated they really needed to be in a hospital setting. |
Dr. Jeremiah Hinson | (37:36) | And then again, in the way that we did for the triage tool, we used risk thresholding to assign specific levels. And so in this case, we assigned 10 levels, 10 risk levels and we called this a Covid-19 deterioration risk level. And then we would make recommendations to the clinicians about whether or not patients should be admitted to the hospital. |
Dr. Jeremiah Hinson | (37:58) | You can see that we derived and tested this thing in over 21,000 visits, we validated it in 16,000 visits, and then we went live with it. An important thing to note is that a lot of the models that were published around Covid-19 and severe Covid-19 at the time relied on looking at patients that we knew had Covid-19. But if you'll recall, we had no idea which patients actually had Covid-19. And so what we did was we put anybody who got tested for Covid-19 into this model and we developed a model for what we called PUIs at the time. |
Dr. Jeremiah Hinson | (38:36) | When we went to the bedside and tried to put this into practice, well, we wanted to put it in a place that made the most sense for when the decision was being made. So this is actually a screen that clinicians go into to decide where the patient's going next. Will they be discharged, admitted, hospitalized? At this time, we were actually also sending patients to a field hospital so they could send them there as well. |
Dr. Jeremiah Hinson | (39:00) | And they would see here a risk level. And so we set a specific risk level and we would say what the risk of 24 hour critical outcome and 72 hour risk for inpatient care needs was. And that would kind of guide their decision. They weren't kind of inundated with all of the information about the model every time, but if they wanted to see more, they would click. If they clicked here, then it would take them to a module where they could see the risk levels, what the specific recommendations were for all of those. And then by drilling down, they could see the panel that I showed you just a couple of minutes ago, or they could even watch a video about how the tool was developed and tested. And they could also send an email from the clinical environment to ask more information if they wanted to follow up. So what we found is that initially people looked at these things, but once they understood how the model was working, they just used it. |
Dr. Jeremiah Hinson | (39:52) | So how did it work? Well, the dotted line here, again, we're going back to those receiver operating characteristics, curves, and you can see that almost always when you derive a model, it works best on the data that you developed it. And we saw that was the case, but even after we went live with the tool, we had two different phases where it was operating live in the background and in another phase where clinicians were actually using it, we found that the performance stayed really high and that it was useful over time and didn't really degrade. |
Dr. Jeremiah Hinson | (40:28) | How did it impact things? Well, we've measured a lot of stuff, I'm showing just a couple of them here. But in the patients who were labeled as high risk, we saw a decrease in the rate of patients who were admitted to a hospital bed and then required an upgrade to the ICU within 24 hours. So this shows is that we were able to identify those patients at high risk of decompensation and get them to the right place on the first decision. |
Dr. Jeremiah Hinson | (40:54) | We also saw a decrease in the rate of mortality within 24 hours in patients who were levels 9 and 10. So 58% decrease in that. There were a lot of things going on at the time, and so likely some other things contributed to both of these outcomes. But this is comparing before and after with the tool. We also saw some slight decreases in length of stay. Discharge rates remain stable, 24 hour returns remain stable as well. So we were able to get more people out of the health system without actually changing the number of people who were coming back. |
Dr. Jeremiah Hinson | (41:28) | So I've told you about tools that operate in several different environments and target different decision points in emergency medicine. So now it's probably useful to talk about what's next. So what do we think is on the horizon for development. So I think three buckets, and there are several more, but these are the three buckets that I think are really important right now. And one of them is how do we make EHR embedded, AI driven clinical decision support that really hits these five targets here. It has to be explainable so that the people who are using it can trust it and know why a recommendation is being given. |
Dr. Jeremiah Hinson | (42:11) | So for the triage tool I gave you example of a patient being recommended for a certain triage level and then we're telling why, right? So we're working at that within our group and in collaboration with Beckman (Coulter) as well, is you make sure that the user understands why they're being told something. The second is making it usable. So I think everybody that works clinically has dealt with interruptive alerts. That's not the way to go. But if we can find a way to make a tool improve our job, the experience of caring for patients and provide better care, that's going to be very, very key. So we're working a lot on usability and there's a lot of research being done in this area as well. |
Dr. Jeremiah Hinson | (42:54) | Making tools trustworthy. So trustworthiness and transparency really kind of come together here. And I think that's about telling people exactly where are these data coming from? Are they data that I can trust? Is the model performing consistently over time? And we talked a little bit about how we can achieve that through ongoing monitoring things. And then really importantly, we have to find a way to implement these tools in a respectful way that makes it very clear that artificial intelligence is not replacing the clinical judgment of nurses, physicians, technicians, anyone. That clinical judgment is super, super important, but it's just another piece of evidence that we can use to support the person providing healthcare at the bedside. |
Dr. Jeremiah Hinson | (43:40) | I think in the near future we're going to see the incorporation of novel diagnostic input into AI models and vice versa. So I think we're going to have models that take a new piece of data. So if you look at what we were doing there with the TriageGO and trying to detect sepsis, it was incorporating a piece of data that is now available through Beckman (Coulter's) hematology analyzers called monocyte distribution width, right? So we can use new pieces of data like that to improve the performance of models, and then we can probably going to be using models to improve the performance of diagnostic tests as well. |
Dr. Jeremiah Hinson | (44:19) | And then the third thing I think we're going to be doing very soon is kind of optimizing and automating the way that we execute diagnostic algorithms. So right now a patient may get screened with one lab, we have to wait for the results of that lab to come back, and then we have to wait for a clinician to look at those results and then another lab may be ordered based on them or another type of diagnostic test. I think in the future, a lot of that's going to be automated by probabilities that are calculated by artificial intelligence. |
Dr. Jeremiah Hinson | (44:49) | So if AI can pass, I don't know if you've seen this, but the ChatGPT tool can pass some of our medical board exams. I think we can develop algorithms that can get us to the right order sets for patients so that when the clinician gets them, they can interpret all the data that are needed. |
Dr. Jeremiah Hinson | (45:08) | So these are references from many of the articles that I talked about here, and I am very happy to answer any questions. If we don't get to a question that you might have during the time we have, I think we have a good amount of time for questions, but if we aren't able to get to it, feel free to email me or if you're interested in collaborating on things, be happy to hear from you too. Thank you. |
Hannah Eichelberger | (45:29) | Awesome. Thanks so much Dr. Hinson, great presentation. And we do have some time for questions here folks, so please feel free to type your questions into the Q&A section on your webinar dashboard and we'll try to tackle as many as we have time for here. So let's take our first question. Can you talk in a little bit more detail about how your sepsis diagnosis tool works and how it compares to existing tools such as NEWS? |
Dr. Jeremiah Hinson | (45:55) | Yeah. So there were two things that I showed. One was a sepsis tool developed by another academic group at a different health system. That tool is using data that are available in the EHR. So things like past medical history, vital signs that have been captured, laboratory data, a pretty complex model, and then it predicts future events. So whether a patient is going to meet criteria for sepsis or not. And it runs it through a different type of model. That was a XGBoosted model, that just different names for different machine learning models. So the one I showed you that builds on our triage model is actually essentially very similar to the model that we described for triage, where we're using Random Forest models. We use the same predictors that you gather at triage, those five simple variables and then some basic labs. And we predict and it far outperforms these other models like NEWS and MUSE and SOFA. Yep. |
Hannah Eichelberger | (47:04) | Got it. Thanks very much. Okay, next question here. Are these tools currently available for use all over the US like an application for an iPhone? And if not, when will they be available to outside entities? |
Dr. Jeremiah Hinson | (47:16) | Yeah, I guess the first answer is yes, with some caveats. So one of the big challenges to a lot of the tools that are developed through research is that it's very hard to make them available outside. So the TriageGO tool that I showed is now available through Beckman (Coulter). So it's a commercial product which allows it to go into any ED anywhere. And the thing that happens prior to implementation of a tool like that is taking data from the local site, retraining an algorithm to ensure that it's working on data from the local population and then actually seeing that it works well and then it can be implemented. So yes, that one is available. |
Dr. Jeremiah Hinson | (48:00) | There are others in the works that a lot of people are working to develop and I think they'll be available soon. So some of those algorithms are not available to the public. So the Covid-19 algorithm for instance, that was one that only was available inside our health system, but the whole group at Beckman (Coulter) is actually working to develop and kind of disseminate these through a commercial pathway. |
Hannah Eichelberger | (48:30) | Excellent. Thanks very much. Next question here, are you taking any splenics into account at all? |
Dr. Jeremiah Hinson | (48:35): | Yeah, so it's a good question. It's a very common question. So we take immunosuppression in general into account, we don't specifically look at a splenic, but they fall into a group of patients that are classified as immunosuppressed. And so that's part of the past medical history elements. So when we're looking at triage, we're really looking for past medical history that has been populated into the Electronic Health Record. And then we use that actually to predict outcome. |
Dr. Jeremiah Hinson | (49:08) | So anecdotally, we've actually seen that the tool can identify patients with some of these kind of high risk conditions who are at higher risk of decompensating than other patients who look essentially the same with that exception and they can often be overlooked. So that's a great question. |
Hannah Eichelberger | (49:26) | Got it. Thanks very much. Okay, couple more questions here and folks please do keep them coming. We have about 10 more minutes, so feel free to continue typing questions into the Q&A section. Next question here; “Really like the AI triage support decision making. Question is, are nurses more often accepting the AI suggestion or overriding it?” |
Dr. Jeremiah Hinson | (49:45) | Yeah, great question. So there's an optimal agreement rate, and so that optimal agreement rate is around 80%. So when a tool is first introduced or before the tool is introduced, the natural agreement rate between the nurse and the tool is somewhere between 50% and 60%. So the decision making definitely changes. When the tool is introduced, the agreement rate tends to be a little lower, maybe you're in the 75% range. But then we find that once it is in steady state, the agreement rate is around 80%. And that's optimal because there's going to be times when a nurse can see something that the algorithm can't see, like the color of a patient, a patient who's very pale, a patient who's not really responding to them and they're getting all the information from a person beside them. An AI driven tool will never be able to see that or won't be able to see that kind of thing in the near future. |
Dr. Jeremiah Hinson | (50:48) | But what we see in increases the kind of agreement rate with it is that we can put in a closed loop learning system. So when there was a disagreement, we can now provide what the outcome was for that patient by following the Electronic Health Record data. And we can tell individual nurses and nurse leadership like, okay, this is a situation where the nurse disagreed with the tool and it was a great catch. This is a situation where the nurse disagreed with the tool and the tool was right, and we probably should have considered making that person a higher acuity or lower acuity. And over time we reach a kind of optimal agreement rate. |
Hannah Eichelberger | (51:25) | Got it. Thanks. Okay, next question here. How important is explainability quotations given that some machine learning models like deep learning may enhance predictive performance at the expense of explainability? |
Dr. Jeremiah Hinson | (51:38) | Yeah, that is a active area of study and debate. The challenge I think is that people want the decisions that they're making to make sense. And so people who are working in this area to achieve explainability are trying to get to a place where even though a decision may have been made that wasn't predicted, people at least want to know what drove it. And so for things like deep learning, the way people are attacking this is by putting machine learning algorithms or other types of algorithms on top of algorithms like deep learning to actually try to discover why a recommendation was being made and then translate that to an explanation for the clinician. |
Dr. Jeremiah Hinson | (52:30) | So I think the answer is that, yeah, there will be times where we may not be able to say physiologically why that particular thing may have happened for a patient, but we should be able to tell the clinician, look, this is why we're giving you the recommendation. So there's a long conversation about interpretability of algorithms versus explainability of algorithms, and I think that's kind of where the answer lies. But I think most people who are making a decision want to know why they're being told to make a decision so they can choose to agree with it or not agree with it. |
Hannah Eichelberger | (53:02) | Got it. Okay, thanks. Next question here. At the moment, only a few devices are able to read monocyte distribution width. What's your opinion about this tool becoming more popular at laboratory machines in the future? |
Dr. Jeremiah Hinson | (53:16) | Yeah, I'll be honest, we've done studies which are also published you can take a look at, that are about monocyte distribution width in particular. And I guess maybe as a researcher and physician, I tend to be skeptical. So when I heard about the tool, I didn't really think it was going to be very useful, but the data bore out that it was quite useful. So the monocyte distribution width is just a marker of variability in the shape and size of a particular lineage of white blood cells. And the reason it's valuable is that it's available for almost every patient in the emergency department. |
Dr. Jeremiah Hinson | (53:58) | So any patient where you have any suspicion of risk for any condition, they're going to get that CBC. There is a current challenge that monocyte distribution width is only available from one manufacturer, which is Beckman (Coulter). And so if you don't have that hematology analyzer, you don't have the ability to get that information. So I guess that'll be kind of up to the individual hospitals as to how highly they value that information and would want to adopt a different hematology analyzer. But I think that the assay itself is valuable as a screening tool and especially when combined with other components on the CBC. |
Hannah Eichelberger | (54:38) | Got it. Okay. And I'm sorry about this, if this is repetition, but we did get a clarifying question. Can TriageGO be trained on data that will include facilities outside of the facility it's used in, especially if performance differs from facility to facility? |
Dr. Jeremiah Hinson | (54:54) | Yeah, so the algorithm is trained on data outside the... so the base is already trained from millions of encounters over time, so it has informed the tool. And then before it goes to a local facility, it is exposed to the data from that facility and retrained on those data and retested on those data. So I think that may be answering the question. If not, I can clarify further. But it learns from everywhere that it's deployed, but then before it's deployed at a specific place, it learns from what's happening at that facility. |
Hannah Eichelberger /td> | (55:30) | Got it. Okay. Thanks for that. Okay, Dr. Hinson, I'm not seeing any other questions at this point in time, so again, I just wanted to thank you for that great presentation and for being online today. Certainly appreciate it. |
Dr. Jeremiah Hinson | (55:43) | Okay, great. Thank you guys. It was nice to be with you. I appreciate the invitation. |